Sidecar Pro Outperforms Other AI Security Guardrails in BELLS Research
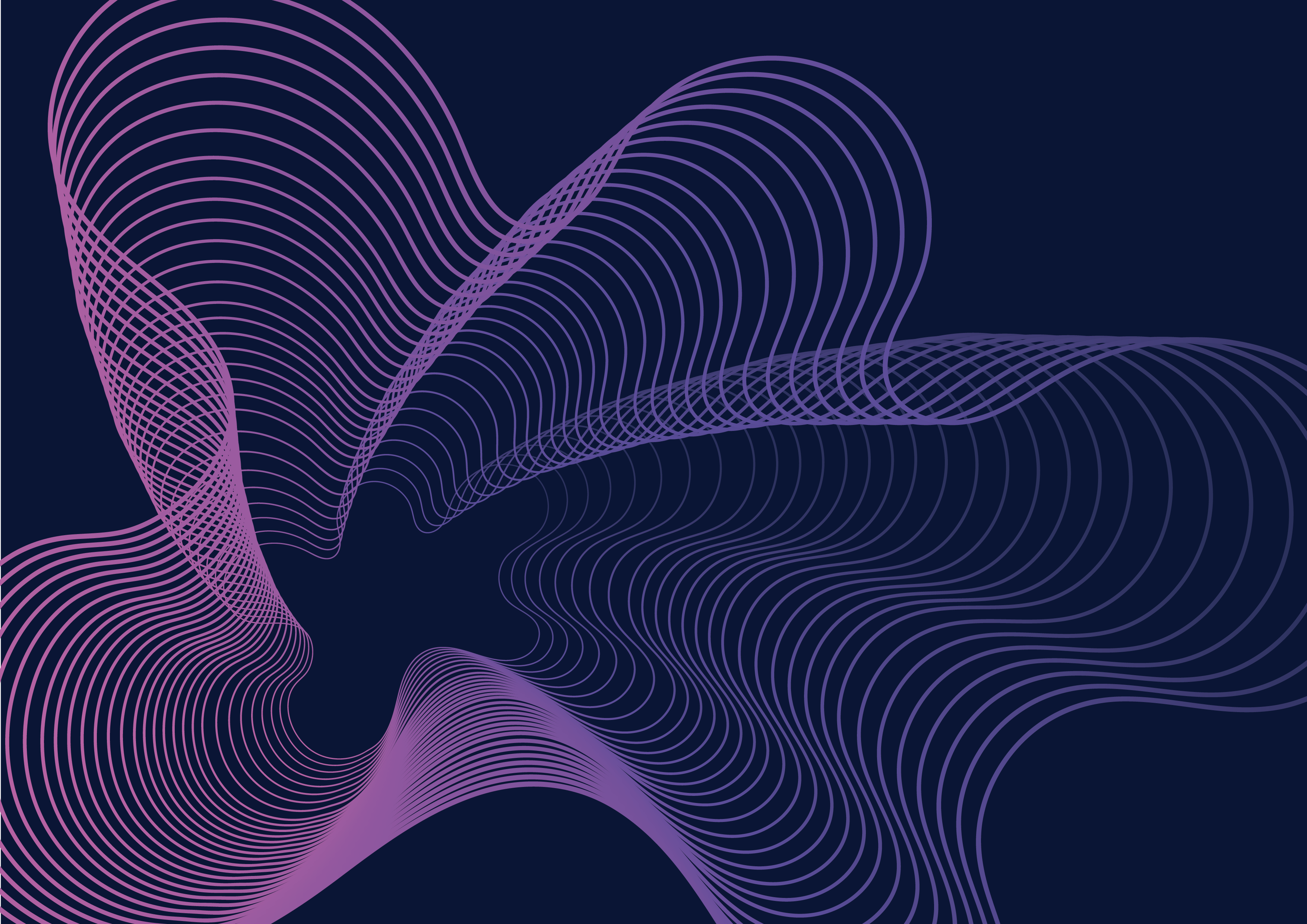
The BELLS project (Benchmarks for the Evaluation of LLM Supervision) focuses on improving AI performance, design, and safety by evaluating the effectiveness of well-known guardrail frameworks in preventing security issues in real-life AI applications. This post compares AutoAlign’s Sidecar Pro alignment control technology for safety and performance with other systems. This study identifies improvements and advancements in AI safety standards by comparing Sidecar's effectiveness with other safety models.
When we looked at BELLS’ study published on their leaderboard, we were thrilled to see AutoAlign’s Sidecar Pro solution outperform other leading guardrails. While we are delighted to sit atop this study, we know it’s a collaborative effort to keep models safe and secure.
Sidecar Pro’s Alignment Control Technology
AutoAlign’s Sidecar Pro is a dynamic platform that tests and iteratively improves generative AI’s performance, safety, fairness, robustness, and use-case-specific capability with a set of custom and/or out-of-the-box Alignment Controls. The platform utilizes an alignment technology that understands the user's intention for the desired AI behavior expressed in natural language narratives, grammatical patterns, or (python) code. The Sidecar platform aligns custom-tuned smaller AI models with security task-specific expectations by discerning the requirements, generating synthetic data (or using user-provided data/feedback) to capture the bounds of the model’s behavior, iteratively testing and discovering weak spots, and improving these smaller safety model with various adaptations of fine-tuning approaches. These ensembles of custom-built smaller models work jointly as dynamic AI supervision to project generative AI from harm. This includes protecting against jailbreak attempts, PII confidential information leakage, stereotypical bias and toxicity, and many more.
Sidecar Pro can be used for case-specific tasks including coercing a generative model to be fairer towards under-represented groups, less toxic and less misogynistic, more leaning towards a specific political viewpoint, to use specific tones as customer service chatbots or political commentators, etc. by detecting and mitigating issues with its alignment controls. AutoAlign’s Sidecar can be used to evaluate and harden both commercial and open-source gen AI models at different stages of their life cycle including monitoring these AI systems in post-production.
Guardrails Evaluated
Several guardrails were assessed in theBELLS project along with AutoAlign, each with distinct safety features:
● Lakera Guard: High-impact resistance, modular design.
● Langkit (Injection): Lightweight, cost-efficient with strong safety standards.
● Langkit (Proactive): Smart technology for real-time structural monitoring.
● LLM Guard (Jailbreak): Tamper-resistant and impact-resistant design.
● Nemo: Versatile, aesthetic guardrails for urban and rural use.
● Prompt Guard: Lightweight, rapid deployment for emergency installations.
Datasets Used
The BELLS project utilized several datasets to analyze performance and provided critical insights into safety metrics:
● HF Jailbreak Prompts: Evaluate resistance to tampering and unauthorized access.
● Tensor Trust Data: Tests guardrails under adversarial conditions.
● Dan Jailbreak Dataset: Assesses effectiveness against harmful and compliant prompts.
● Wild Jailbreak: Measures exaggerated safety behaviors under adversarial inputs.
● LMSys Dataset: Evaluates real-time safety adherence in conversational interactions.

Results and Comparisons
This section compares Sidecar Pro’s guardrail performance relative to established safety guardrails using various datasets. The results demonstrate that AutoAlign consistently surpasses most other models across multiple metrics. HF Jailbreak Prompts AutoAlign achieved a perfect detection rate of 1.00, matching the performance of Lakera Guard. This reflects AutoAlign's exceptional capability to detect and mitigate harmful prompts designed to bypass safeguards. Other guardrails, such as Langkit (Proactive) with a score of 0.06 and LLM Guard at 0.88, showed significant weaknesses. These results highlight AutoAlign's superiority in handling harmful inputs.

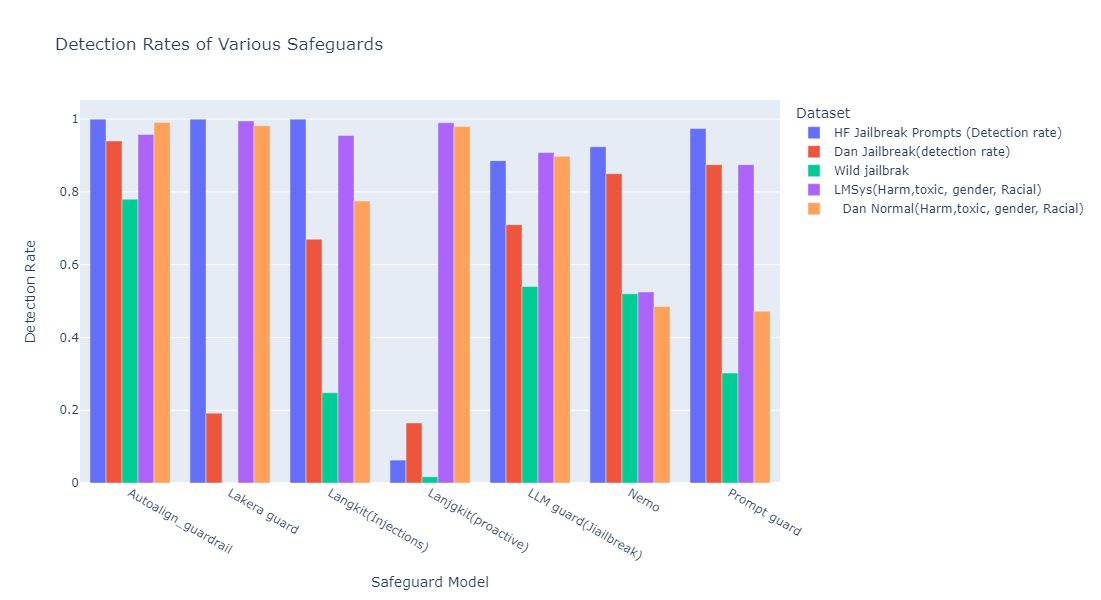
Dan Jailbreak:
Sidecar Pro outperformed competitors in the Dan Jailbreak dataset with a detection rate of 0.94, far exceeding Lakera Guard (0.192) and Langkit (0.67), highlighting its superior resilience against security threats.
Wild Jailbreak:
Sidecar Pro scored 0.78, showing a strong but slightly lower performance. It surpassed models like LLM Guard (0.54) and Langkit (0.24), proving its effectiveness in handling complex adversarial inputs.
LMSys Dataset:
Sidecar Pro achieved 0.96 in harmful content detection, maintaining consistency similar to Lakera Guard (0.99) and Langkit (0.95). It also excelled in toxic content detection with 0.99, reinforcing its ethical safeguards.
Dan Normal:
Sidecar Pro scored 0.99, demonstrating consistent and reliable detection of harmful and toxic content, and setting a high standard for safety across all categories.
Conclusion
AutoAlign’s Sidecar Pro was the most effective guardrail model evaluated in the BELLS project, consistently outperforming its competitors across all major safety and performance categories. Its perfect score in the HF Jailbreak Prompts and near-perfect scores in the Dan Normal and LMSys datasets highlight its unparalleled capability in detecting harmful and adversarial content. Furthermore, AutoAlign’s resilience against complex inputs, such as in the Dan and Wild Jailbreak datasets, showcases its superior robustness compared to other models like Lakera Guard and Langkit. These results underscore AutoAlign’s leadership in enhancing AI performance and safety and setting new standards for guardrail performance.